So You Want to Build an AI Company?
Sorry, but there’s no AI for building an AI company (yet)
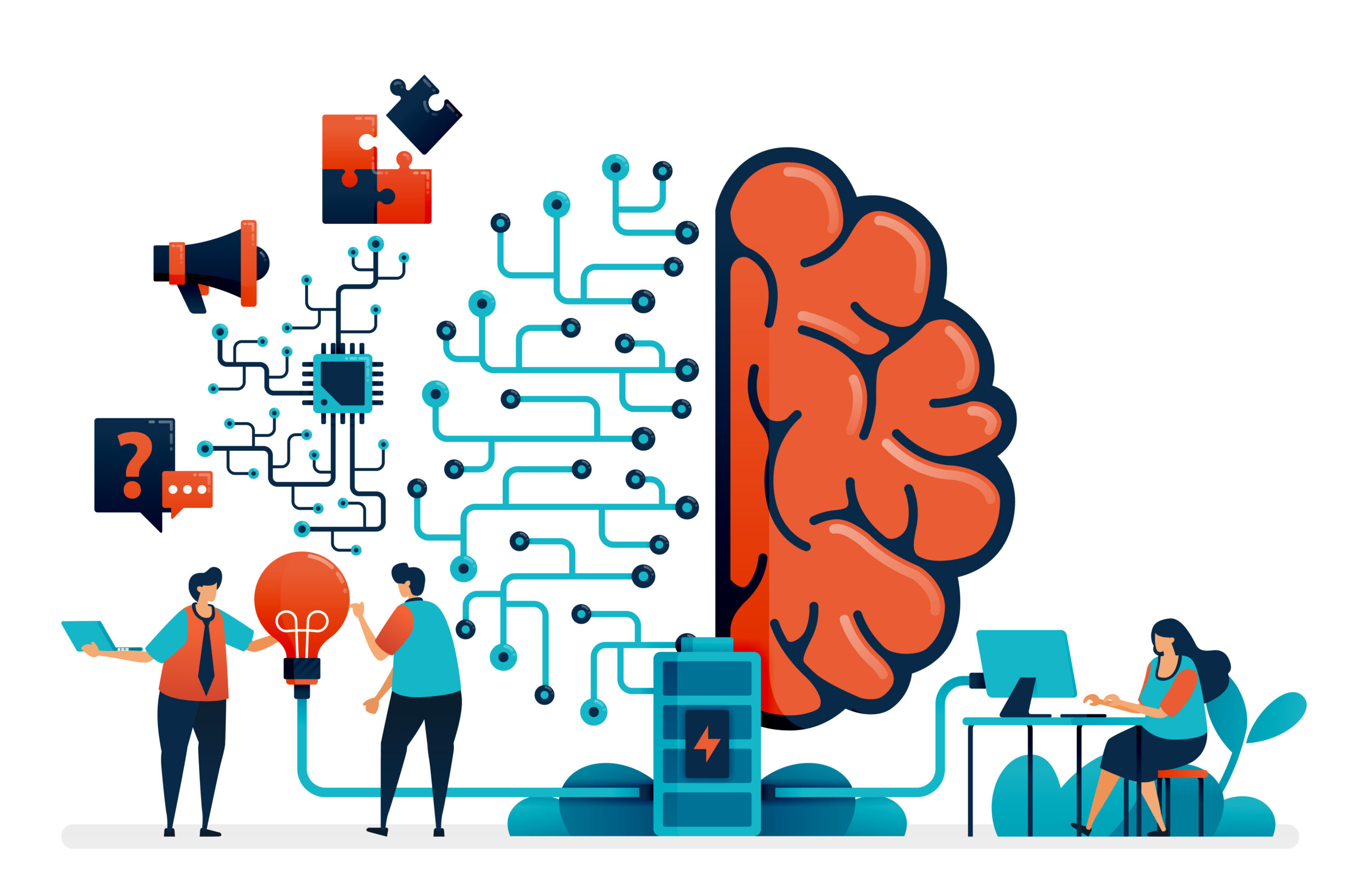
Sorry, but there’s no AI for building an AI company (yet)
AI for tax evasion. AI for dry cleaning. AI for shucking corn.
“AI for X” is the new “Uber for X.” It’s not a hot take to say AI and GenAI startups are coming out of our ears. Run a Google News search for AI company and see how many use cases you can cross out on your “AI for X” bingo card. Or follow the money: In the US, VC funding for AI totaled $290B over the past five years.
AI is catnip for some VCs. And why not? A charismatic, whip-smart founder essentially pitches something like, “When you fund us, we’ll solve this broad but incredibly ambitious problem. Further, we’ll create a huge, proprietary dataset, then build a valuable, predictive, efficacious model on top of this data.”
Cha-ching.
However, building an AI company is not that simple. Many investors overestimate how many teams out there can pull it off, overlook factors like timing and luck and just how long it takes for a data company to get off the ground. After all, let’s not kid ourselves, if you’re investing in an AI company you’re really investing in a data company. Other AI-trepreneurs might tout a “reskinning” of OpenAI or another competing GenAI company. While this might generate quicker results, the low barrier for entry means countless others are doing the same, probably at lower costs.
The path to building an AI company is long and daunting. In no specific order, here are the steps prospective AI founders can expect to take, likely over the course of several years.
1. Lots of data, lots of scale
It goes without saying, but any AI company needs a Great Barrier Reef full of data to stay competitive.
Does this data already exist, or do you need to generate it? If the former, who’s collecting it and therefore owns it, for what purpose, and is it scalable? Grabbing data from a single source is a risky proposition, but the alternate route—working with competing data providers—runs the risk of uniqueness dilution. Tough sledding either way.
In Deduce’s case, we knew from the get-go that our real-time fraud prevention solution required access to every online US consumer, across a broad range of online activities and devices. We scoured the internet for pinch points, such as SDK and JavaScript deployments, that reported consumer authentication events like account creation, log in, forgot password, online comments, and more. It wasn’t overnight, but we got the scale we needed: 150K websites and applications (and growing).
2. Get your copyright ducks in a row
If you train your AI models using copyrighted data, you will be sued up the wazoo. This is precisely why OpenAI struck deals with Reddit and News Corp earlier this year, granting them access to data from Reddit boards, NYT and WSJ articles, among other content. And more recently, Sono and Udio are being sued by the record labels for copyright infringement. The last thing a VC wants is to line the pockets of lawyers that aren’t helping grow the business they’ve invested in. Worse, cease and desist notices are flying and that’s a bad day for your capital partners.
Consider hiring a data licensing team to stay in the clear. Navigating legislation like the NO FAKES Act and Generative AI Copyright Disclosure Act is no easy task, especially with trade associations and the federal government helping to enforce such measures.
Without data copyright experts in your bullpen, good luck tracking and reporting licensed data, in addition to the other tasks required to ensure compliance.
3. GDPR-oblems, CCPA-ndemonium
Unfortunately, the NO FAKES And Generative AI Copyright Disclosure Acts aren’t the only beasts inside the AI Compliance Thunderdome. AI companies also must tussle with the big bad GDPR (General Data Protection Regulation) and CCPA (California Consumer Privacy Act).
As of May 2024, companies including Google, Amazon, and Meta are responsible for more than 2,000 violations of GDPR alone, triggering over €4.5B in fines. If the tech behemoths are struggling with data privacy compliance, then AI upstarts have their work cut out for them.
4. Beef up your infrastructure
Featherweight infrastructure won’t cut it in an AI-eat-AI world. And advancing to the upper echelon of infrastructural weight classes is a slow burn.
At Deduce, for example, the 99.5% accuracy of our real-time trust scores is the product of an identity graph we built and perfected over the course of five years. There’s no cheat code for building and deploying software that ingests and stores over 1.5B daily identity events from more than 150K sites and apps.
Amassing the infrastructure needed for this level of ingestion and storage—while navigating global data privacy rules—is likely a multi-year exercise. In fact, it may be downright unrealistic for companies outside of the FAANG Gang.
Sure, you could outsource your cloud computing and storage needs to AWS, Azure, and the like. But the premium services these vendors deploy—such as NVIDIA-powered AI computing power—are pricey, and as competition for these resources increases this will become a significant expense.
5. Data redundancy, testing, and monitoring
Establishing data redundancy, and performing effective testing and monitoring, is crucial. Otherwise, data partners, customers, and the channel are unlikely to grant access to critical paths, namely account creation, log-in, and checkout workflows (in the case of e-commerce).
Again, not a breezy process. Testing, alone, poses many challenges. Take test data, for instance. Algorithms need ample data to learn, but gathering enough data—data that’s relevant to the actual scenarios the algos will face—is tough.
There’s also the challenge of testing for biases, plus the issue of interpretability: What exactly caused the system to make Determination A instead of Determination B? And don’t forget the task of continually testing models over time to account for new data.
6. The data pipeline: normalize, dedupe, cleanse
Impactful AI companies rely on A+ data pipelines. These pipelines are the horsepower for big data predictive analytics, allowing for data access, data formatting, and the activation of data workflows. They also assist with normalizing, deduping, and cleansing.
However, the path to a potent data pipeline is a circuitous one. A mere few of the obstacles facing AI companies:
- Complexity. Implementing data from various sources, and handling numerous pipeline stages, is tricky. Poor visibility hinders the observation of pipeline behavior.
- Quality. Low-quality data mucks up models and hinders decision-making. It’s difficult to sustain a high quality of data in every part of a pipeline data flow.
- Scalability. Data pipelines must scale alongside data volumes to maximize throughput. An inability to do so leads to costly logjams.
7. Predictive analytics models
Predictive analytics models leverage real-time data to help AI companies make the best possible business decisions, but to successfully deploy these models you’ll need all the help you can get.
On the data end of things, there is no shortage of potholes. Inconsistent data, overly dirty data, old data, poorly labeled data. The dataset(s) used to train your models can be too small; then again, overfeeding your models wastes time and resources.
With models, too much complexity can hamper efficacy and their ability to monitor, adapt, and respond to evolving threats. Algorithm and feature selection, and, later, model evaluation, further complicate deployment and the effectiveness of predictions.
8. Find data partners to test, measure, and balance models
Staying neck-and-neck with your AI rivals is much easier with help from data partners. These partnerships provide access to a larger breadth of data and valuable customer and market-specific insights.
Still, there are many boxes to tick. Is the data being shared accurate, dependable, safe? Is the data being exchanged between both organizations compliant with privacy and security regulations? Collaborating with a data partner is even harder across time zones or countries, especially if expectations for the partnership aren’t sufficiently communicated at the start.
The privacy-compliance tightrope act involved in these partnerships takes a lot of elbow grease, time, and scrutiny. Look at the partnerships between Microsoft/Apple and OpenAI. Is the data from ChatGPT users on Apple devices, for example, bidirectional—is it delivered to OpenAI, given all of the ensuing privacy and compliance issues? Is this truly a consortium model where all boats rise higher with every interaction?
Bottom line: There are miles and miles of red tape in data sharing land, making data partnerships—beneficial (and necessary) as they may be—difficult to land.
9. Securing “crown jewel” data
In the same vein of data partnerships, it behooves AI companies to pursue “crown jewel” data from their customers. This real-time and scalable data, for Deduce, takes the shape of first- and third-party fraud data, chargeback and charge-off data, etc.
These agreements don’t materialize without significant legal negotiation and economic consideration. Data privacy agreements are a knotty, fine print-laden mess. Oh yes, and it’s the result of months of sales activity and account nurturing to demonstrate business impact value to the company you are selling to. And the legal weeds are deep. Will you be a controller or a processor of data? Their compliance and privacy people will surely run you through the ringer.
Why? Because the companies from which you’re receiving data have privacy relationships with their customers that must be respected by anyone they do business with. Many of the data breaches at the top of your news feed are perpetrated by vendors and partners, though it’s the consumer brand that ultimately takes the blame.
10. Refinement, feedback, and real-time efficacy
Data is always changing. New data is spawning as I’m typing these very words. Continually refining your data is imperative and, yes, very tedious. In fact, much of the lengthy refinement process will need to be carried out multiple times before any positive results are generated.
Perhaps the most obvious mother lode of refined data is doing your own sourcing. The use of bots, even clever ones that mimic real human behavior, to access individual pages on a website and capture all the information, is rarely permitted in any terms and conditions of use. There’s also the option to buy refined data from a provider. However, both of these options may not be options at all for companies lacking the necessary expertise and resources, legal included.
If you’re a smaller AI enterprise traversing the bumpy road to data refinement, feedback data, and real-time efficacy, enjoy the scenery. It’s gonna take a while.
The downfall of AI companies: blurry vision
My biggest piece of advice for companies joining the AI fracas? Get your vision checked.
Building a successful AI company ultimately starts with the right vision. Are you solving a bounded problem for enterprises? Society at large? Is it a specific problem that can be realistically addressed?
You need to have a specific value-add in mind, and it must have commercial appeal. Too many AI companies lack specificity around what they’re looking to solve; instead they parrot the OpenAI/ChatGPT approach of trying to be everything to everybody.
This is my third AI startup (we previously dared to use “ML”), and building Deduce wasn’t any easier than the other two. The specific problem we set out to solve was identity intelligence. It took almost five years and nearly $30M in funding (pennies compared to other AI startups) to build an identity graph that protects some of the largest organizations in the world. 185M unique identities—essentially the entire US online population—observed multiple times every week across a broad range of activities in service of shoring up the new account-opening flow.
Deduce’s recency and frequency of online activity observation, coupled with AI-driven pattern recognition, roots out the fake humans tormenting finservs and universities, interfering in elections, and irreparably harming many other facets of society. Good users get authenticated FAST, bad ones don’t.
The point I’m making is this wasn’t a vision that a couple of engineers and I cooked up in some basement and brought to fruition over the course of a few months, let alone a few years. Compiling the requisite data, scale, and infrastructure, in addition to the other minutia outlined above, to reach this point, was a capital-G Grind.
Above all else, understand this: building an AI company is a marathon—and it’s a 50K, not a 5K.
Shortcuts? They don’t exist. “AI for X” may be in vogue, but there is no AI for building an AI company (yet).